Run full timeseries simulations
In this section, we will learn how to:
run full timeseries simulations using the
PVEngine
class, and visualize some of the resultsrun full timeseries simulations using the
run_timeseries_engine()
function
Imports and settings
[1]:
# Import external libraries
import os
import numpy as np
import matplotlib.pyplot as plt
from datetime import datetime
import pandas as pd
import warnings
# Settings
%matplotlib inline
np.set_printoptions(precision=3, linewidth=300)
warnings.filterwarnings('ignore')
# Paths
LOCAL_DIR = os.getcwd()
DATA_DIR = os.path.join(LOCAL_DIR, 'data')
filepath = os.path.join(DATA_DIR, 'test_df_inputs_MET_clearsky_tucson.csv')
Get timeseries inputs
[2]:
def export_data(fp):
tz = 'US/Arizona'
df = pd.read_csv(fp, index_col=0)
df.index = pd.DatetimeIndex(df.index).tz_convert(tz)
return df
df = export_data(filepath)
df_inputs = df.iloc[:24, :]
[3]:
# Plot the data
f, (ax1, ax2, ax3) = plt.subplots(1, 3, figsize=(12, 3))
df_inputs[['dni', 'dhi']].plot(ax=ax1)
df_inputs[['solar_zenith', 'solar_azimuth']].plot(ax=ax2)
df_inputs[['surface_tilt', 'surface_azimuth']].plot(ax=ax3)
plt.show()
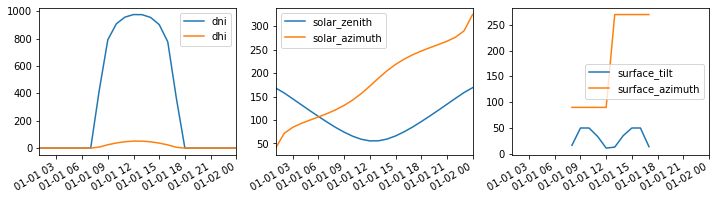
[4]:
# Use a fixed albedo
albedo = 0.2
Prepare PV array parameters
[5]:
pvarray_parameters = {
'n_pvrows': 3, # number of pv rows
'pvrow_height': 1, # height of pvrows (measured at center / torque tube)
'pvrow_width': 1, # width of pvrows
'axis_azimuth': 0., # azimuth angle of rotation axis
'gcr': 0.4, # ground coverage ratio
'rho_front_pvrow': 0.01, # pv row front surface reflectivity
'rho_back_pvrow': 0.03, # pv row back surface reflectivity
}
Run single timestep with PVEngine and inspect results
Instantiate the PVEngine
class and fit it to the data
[6]:
from pvfactors.engine import PVEngine
from pvfactors.geometry import OrderedPVArray
# Create ordered PV array
pvarray = OrderedPVArray.init_from_dict(pvarray_parameters)
# Create engine
engine = PVEngine(pvarray)
# Fit engine to data
engine.fit(df_inputs.index, df_inputs.dni, df_inputs.dhi,
df_inputs.solar_zenith, df_inputs.solar_azimuth,
df_inputs.surface_tilt, df_inputs.surface_azimuth,
albedo)
The user can run a simulation for a single timestep and plot the returned PV array
[7]:
# Get the PV array
pvarray = engine.run_full_mode(fn_build_report=lambda pvarray: pvarray)
# Plot pvarray shapely geometries
f, ax = plt.subplots(figsize=(10, 3))
pvarray.plot_at_idx(15, ax, with_surface_index=True)
ax.set_title(df.index[15])
plt.show()
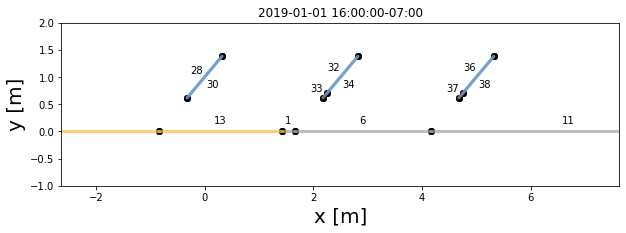
The user can inspect the results very easily thanks to the simple geometry API
[8]:
# Get the calculated outputs from the pv array
center_row_front_incident_irradiance = pvarray.ts_pvrows[1].front.get_param_weighted('qinc')
left_row_back_reflected_incident_irradiance = pvarray.ts_pvrows[0].back.get_param_weighted('reflection')
right_row_back_isotropic_incident_irradiance = pvarray.ts_pvrows[2].back.get_param_weighted('isotropic')
print("Incident irradiance on front surface of middle pv row: \n{} W/m2"
.format(center_row_front_incident_irradiance))
print("Reflected irradiance on back surface of left pv row: \n{} W/m2"
.format(left_row_back_reflected_incident_irradiance))
print("Isotropic irradiance on back surface of right pv row: \n{} W/m2"
.format(right_row_back_isotropic_incident_irradiance))
Incident irradiance on front surface of middle pv row:
[ nan nan nan nan nan nan nan 117.633 587.344 685.115 652.526 616.77 618.875 656.024 685.556 550.172 87.66 nan nan nan nan nan nan nan] W/m2
Reflected irradiance on back surface of left pv row:
[ nan nan nan nan nan nan nan 8.375 6.597 39.275 58.563 68.346 64.176 47.593 32.984 25.216 7.044 nan nan nan nan nan nan nan] W/m2
Isotropic irradiance on back surface of right pv row:
[ nan nan nan nan nan nan nan 0.076 2.15 3.116 1.697 0.199 0.414 2.627 4.208 2.83 0.066 nan nan nan nan nan nan nan] W/m2
Run multiple timesteps with PVEngine
The users can also obtain a “report” that will look like whatever the users want, and which will rely on the simple geometry API shown above. Here is an example:
[9]:
# Create a function that will build a report
from pvfactors.report import example_fn_build_report
# Run full simulation
report = engine.run_full_mode(fn_build_report=example_fn_build_report)
# Print results (report is defined by report function passed by user)
df_report = pd.DataFrame(report, index=df_inputs.index)
df_report.iloc[6:11]
[9]:
qinc_front | qinc_back | iso_front | iso_back | |
---|---|---|---|---|
2019-01-01 07:00:00-07:00 | NaN | NaN | NaN | NaN |
2019-01-01 08:00:00-07:00 | 117.632919 | 9.703464 | 5.070103 | 0.076232 |
2019-01-01 09:00:00-07:00 | 587.344197 | 4.906038 | 12.087407 | 2.150237 |
2019-01-01 10:00:00-07:00 | 685.115436 | 33.478098 | 17.516188 | 3.115967 |
2019-01-01 11:00:00-07:00 | 652.526254 | 52.534503 | 24.250780 | 1.697046 |
[10]:
f, ax = plt.subplots(1, 2, figsize=(10, 3))
df_report[['qinc_front', 'qinc_back']].plot(ax=ax[0])
df_report[['iso_front', 'iso_back']].plot(ax=ax[1])
plt.show()
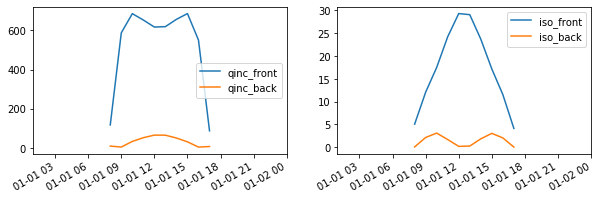
'qinc'
) on the back surface of the rightmost PV row. A good way to get started building the reporting function is to use the example provided in the report.py
module of the pvfactors package.[11]:
def new_fn_build_report(pvarray): return {'total_inc_back': pvarray.ts_pvrows[1].back.get_param_weighted('qinc')}
Now we can run the timeseries simulation again using the same engine but a different report function.
[12]:
# Run full simulation using new report function
new_report = engine.run_full_mode(fn_build_report=new_fn_build_report)
# Print results
df_new_report = pd.DataFrame(new_report, index=df_inputs.index)
df_new_report.iloc[6:11]
[12]:
total_inc_back | |
---|---|
2019-01-01 07:00:00-07:00 | NaN |
2019-01-01 08:00:00-07:00 | 9.703464 |
2019-01-01 09:00:00-07:00 | 4.906038 |
2019-01-01 10:00:00-07:00 | 33.478098 |
2019-01-01 11:00:00-07:00 | 52.534503 |
[13]:
f, ax = plt.subplots(figsize=(5, 3))
df_new_report.plot(ax=ax)
plt.show()
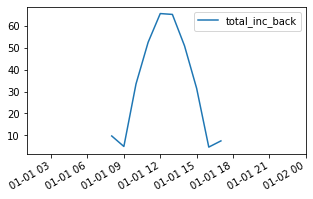
Run one or multiple timesteps with the run_timeseries_engine()
function
run.py
module of the pvfactors package.[14]:
# import function
from pvfactors.run import run_timeseries_engine
# run simulation using new_fn_build_report
report_from_fn = run_timeseries_engine(new_fn_build_report, pvarray_parameters, df_inputs.index,
df_inputs.dni, df_inputs.dhi,
df_inputs.solar_zenith, df_inputs.solar_azimuth,
df_inputs.surface_tilt, df_inputs.surface_azimuth,
albedo)
# make a dataframe out of the report
df_report_from_fn = pd.DataFrame(report_from_fn, index=df_inputs.index)
[15]:
f, ax = plt.subplots(figsize=(5, 3))
df_report_from_fn.plot(ax=ax)
plt.show()
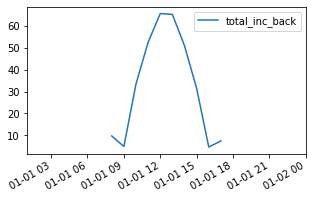
The plot above shows that we get the same results as previously.